Synergizing Knowledge Graphs with Large Language Models (LLMs): A Path to Semantically Enhanced Intelligence
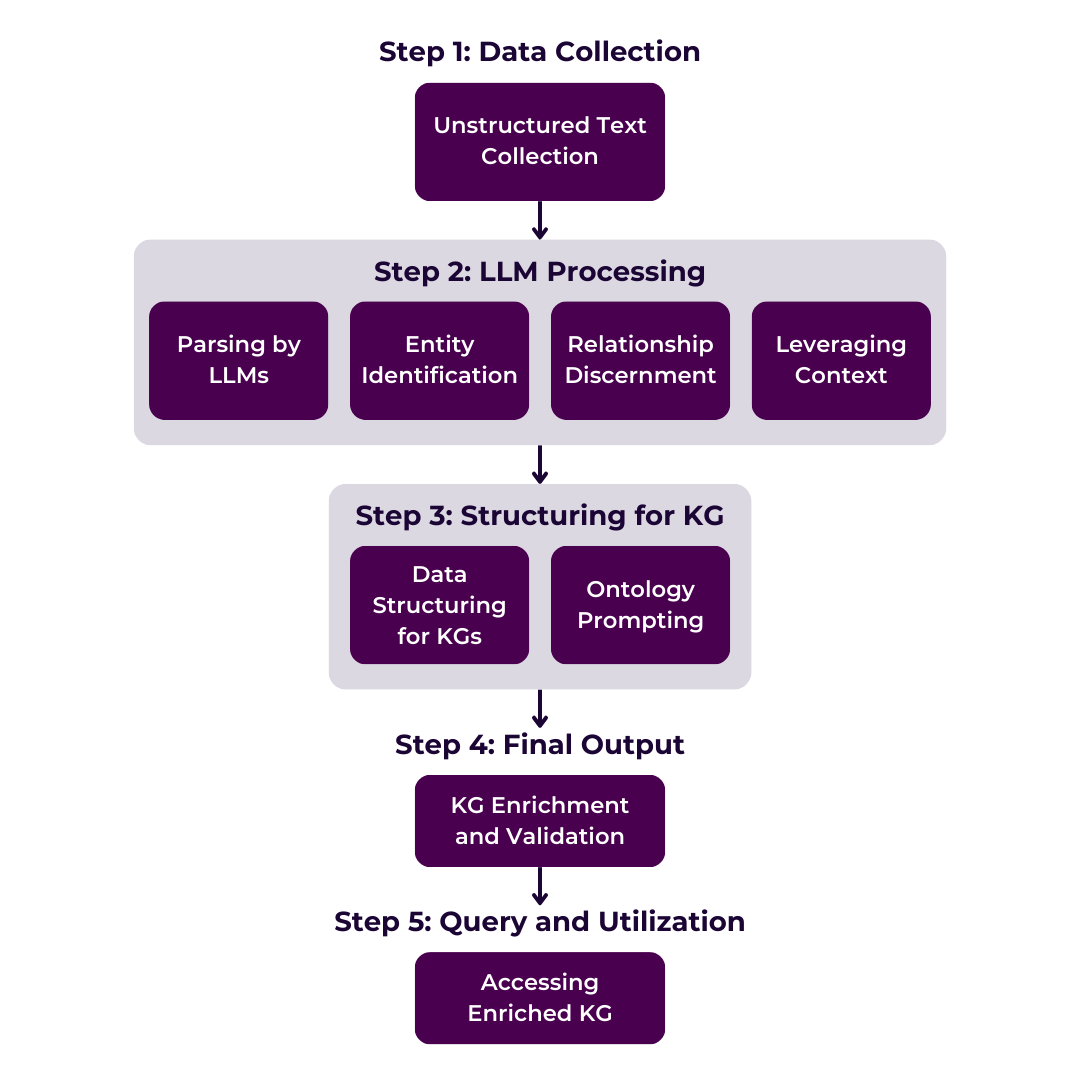
Why do Large Language Models (LLMs) sometimes produce unexpected or inaccurate results, often referred to as ‘hallucinations’? What challenges do organizations face when attempting to align the capabilities of LLMs with their specific business contexts? These pressing questions underscore the complexities and potential problems of LLMs. Yet, the integration of LLMs with Knowledge Graphs (KGs) offers promising avenues to not only address these concerns but also to revolutionize the landscape of data processing and knowledge extraction. This paper delves into this innovative integration, exploring how it shapes the future of artificial intelligence (AI) and its real-world applications.
Introduction
Large Language Models (LLMs) have been trained on diverse and extensive datasets containing billions of words to understand, generate, and interact with human language in a way that is remarkably coherent and contextually relevant. Knowledge Graphs (KGs) are a structured form of information storage that utilizes a graph database format to connect entities and their relationships.