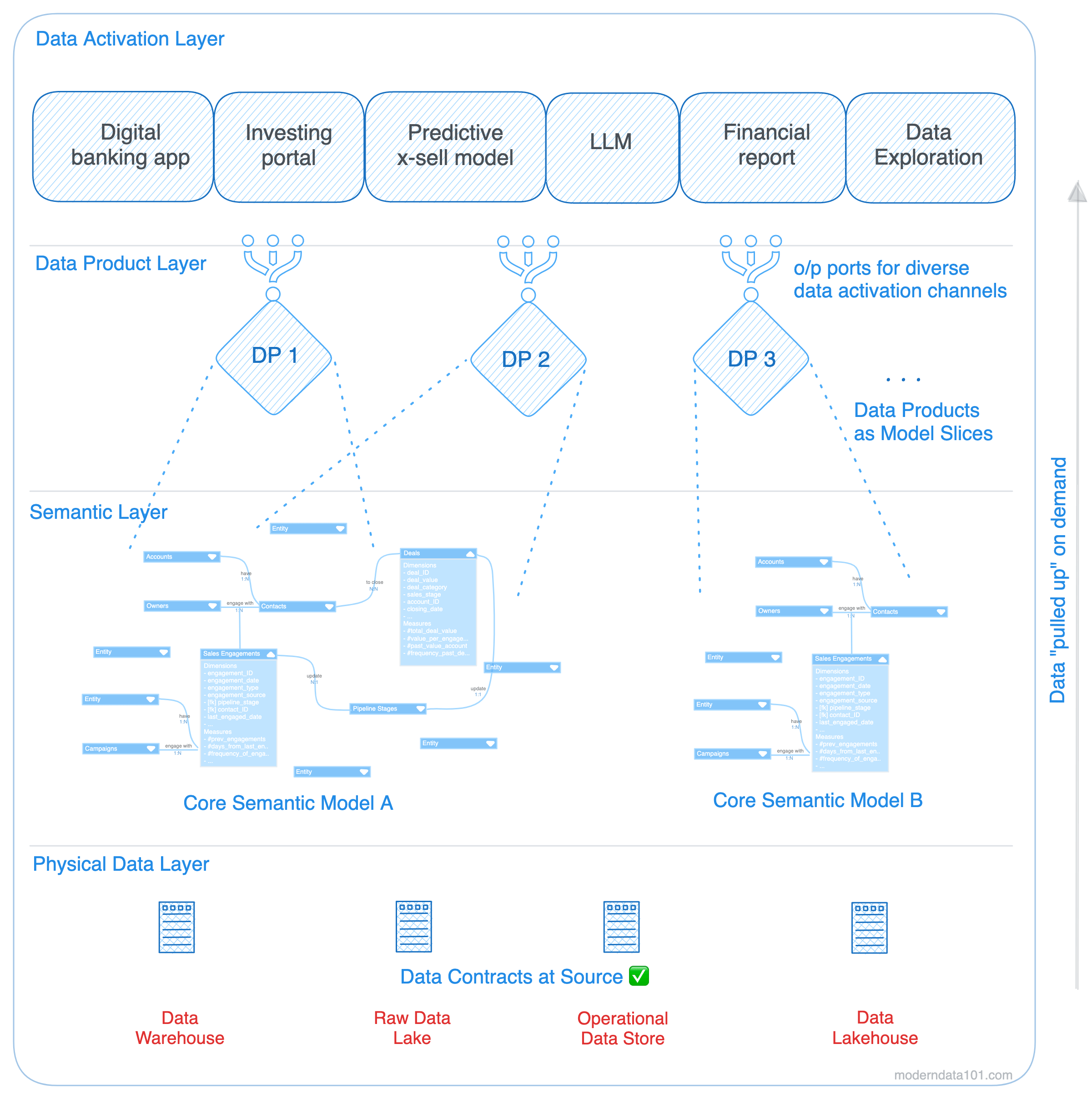
Before you dive in, in the interest of saving your time, we want to call out that this piece is a little longer than usual since it focuses on some features, limitations, and solutions of the tool at hand. You can view the points we’ve covered at a glance in the TOC below. This read is ideally suited for data leaders or data engineering leads who are focusing on optimising their dbt investments and want to enhance either of:Cost savingsData monetisation effortsOverall experience of users and data consumersIf you’re new here, feel free to refer to the previous parts in this series on Leveraging Existing Stacks/Tools for Data Product Builds:Part 1: Cost-optimisation with Data Products on SnowflakePart 2: Snowflake for Data Products: Data Monetisation & ExperienceTable of Contents (TOC)Introduction & ContextThe Need to Shift Conversations from ETL to Data Products + Gaps in dbtData Products: One of many outcomes of Self-Service Platforms, but an Important OneHow to Leverage Your Existing Stack (with dbt) to Build Data ProductsCost SavingsLarge dbt Models May Lead to High Compute CostsInfrastructure CostsMaintenance, Support, & Operational CostsIncreasing Appetite for RevenueScale & PerformanceHow transformations/ETL gains a new stage and is ready for scaleEnhancing Experience for All (customers & business operatives)Jumping In ⬇️Undeniably, dbt is a data developer’s best friend. A product is known for how easily it changes the lives of its users, and dbt has done it without fail. There was a time when transformations used to be a big task and bottleneck for centralised data engineering teams.